The Fed's dangerous gamble: The robust data hides an economic divide
Original author: ◢ J◎e McCann, founder of Asymmetric
Original translation: Deep Tide TechFlow
(The following content was originally published in the macro section of our August Asymmetric Market Update™️, which you can subscribe to for free here)
In our previous Macro Commentary, we focused on key topics related to potential market impact, the global situation and how to navigate these complex times.
We discussed (a month before the bank panic surfaced and scared the markets) the risks faced by small and medium-sized banks due to the uneven distribution of excess reserves, despite the large amount of reserves in the system.
We repeatedly mentioned mixed economic data and discussed the concept of “duck economics”: Everything may seem to be going well on the surface, but there is a lot going on in the dark. Beauty is in the eye of the beholder. While the headline economic data is strong, if you dig deeper you can weave any narrative you prefer, bullish or bearish.
We also analyzed the Magnificent Seven versus the rest of the stock market. Similar to the economic data, the stock indices performed well; but a deeper look revealed that the stocks of the Magnificent Seven performed extremely well while the rest of the market was flat or even declining.
In this episode of Asymmetric Macro, we will tie together all of the concepts discussed previously into a coherent story, starting and ending with the theory of monetary policy itself.
Monetary Policy
For any data set, you need to define the underlying distribution before you can do any meaningful analysis. To simplify the description, we will use three basic distributions. While none of them are perfect, the point will be clear. Headline economic data are used to describe the overall or average economy, which is conceptually sound because you can't tailor economic policy to each individual (to take an extreme example). From many perspectives, this is "unfair" and unimplementable in reality. Therefore, we use aggregate data to describe the state of the economy and thus determine the most appropriate monetary policy for that aggregate data. Let's first understand the three types of distributions to describe the underlying population.
Note: We are not writing a PhD thesis. This discussion is not complete or foolproof because we have limited space. We have woven a story that is intimately connected to the current state of the world and economic policy. So rather than nitpicking over trivial details, let’s think about these concepts and their potential impact at a mental level.
Uniform Distribution
Figure: Uniform Distribution
As you can see, a uniform distribution is one where every observation (in this case, the socioeconomic status of an individual) is the same. A uniform distribution would be the communist ideal. A uniform distribution would also produce the best dataset to conduct monetary policy analysis on. If everyone was in the same position, there would be no variance, so the “average data” would represent everyone perfectly. Therefore, monetary policy based on this data would be perfect (assuming economic theory is valid and applied strictly as a rule). We know this is not the case. Communist ideals are often difficult to achieve.
Normal Distribution
Figure: Normal Distribution
In a normal distribution, the mean, median, and mode are identical. Exactly half of the observations (in this case, the socioeconomic status of individuals) are to the right of the center, and the other half are to the left of the center. The distribution implies that socioeconomic density is highest near the mean, with the number of privileged or disadvantaged individuals decreasing as deviations from the mean increase. Even “average data” can work with a dominant middle class and a reasonable distribution of wealth (like the US which was more balanced in the not-too-distant past than it is today). While it’s not perfect, the density is still concentrated around the mean, so monetary policy based on this data is reasonable because it captures the situation of most of the population (although monetary policy is not relevant at the ends of the population; in a normal distribution, that’s a relatively small fraction).
Bimodal Distribution
Figure: Bimodal Distribution
A bimodal distribution is one in which there are two modes. In other words, the results of two processes with different distributions are combined in one set of data.
This bimodal feature has been popping up a lot lately in all aspects of our world. Let's look at some of the relevant examples we mentioned earlier.
Excess Bank Reserves Are Not Evenly Distributed
In Asymmetric’s February 2023 release, we mentioned that “while there are abundant excess reserves in the system, they are not evenly distributed. These reserves are concentrated in money center banks (like JPM, etc.)”
So, despite the fact that the aggregate amount of excess reserves was very ample, we had a banking crisis that resulted in the Fed having to set up an emergency funding facility to support many banks that did not have sufficient reserves. Several major banks had already collapsed before this facility was opened. Why did this come as a surprise? Because the data on excess reserves is superficial and does not take into account the actual distribution of these reserves. Many banks have no reserves, while some banks have most of the reserves. This is a bimodal distribution. Aggregate data alone does not accurately reflect the true situation in the banking industry. Therefore, the distribution here is critical but has been overlooked.
The uneven distribution of reserves and the subsequent emergency funding facility resulted in weak banks having to pay large interest charges to maintain their balance sheets and grow deposits. And the strong banks (like JPM) get a nice interest income on their excess reserves. It’s like “transferring wealth from the poor to the rich”. One could argue that this is a penalty for poor management, and that’s not wrong. But it still leaves you with a bimodal distribution going forward. And it’s becoming increasingly bimodal given the dynamics.
Small vs. Giant Companies
In Asymmetric’s July 2024 update, we published the following chart:
Chart: Magnificent Seven vs. 493 Other Companies, SP 500 and Russell 2000
Looking at the Magnificent Seven vs. the rest of the stock market (particularly the Russell) also shows a somewhat bimodal distribution. You have a group of large companies that are doing very well; and then you have a group of smaller companies that are not nearly as successful as these giants.
One could argue that this is a capitalist result of creative destruction, and that’s not wrong (we’ll ignore the effects of monopoly/oligopoly industries for this discussion). Regardless, given the current dynamics, this still leaves you with a bimodal distribution in the future that is still getting worse (or a series of monopolies at the boundary).
Some of these results can be attributed to the scalability of technology. Once you dominate a field, you drain business potential and capital from your competitors. As a result, these large companies end up accumulating a lot of cash and making record profits. They buy back their shares and earn a lot of interest income on this cash. The smaller companies, on the other hand, are more heavily in debt (not rich) and have to pay a lot of interest to survive. It’s like a “wealth transfer from the poor to the rich”.
Socioeconomic Distribution
We have chosen the following chart as a convenient example of a bimodal distribution in socioeconomic status. This data set has two different modes, representing the fragmentation of society. Is it useful to look at the average credit score here? Not at all. That is exactly the point. We are used to looking at average data, but in a bimodal distribution this can be at the minimum unhelpful and at the most very harmful and misleading to the analysis.
Figure: Socioeconomic Distribution of High Credit Scores
We can add more details around the distribution of personal savings, debt/credit service charges, etc., but we all know what it will show: a bimodal distribution. As the above example shows, those who pay high interest charges are facing huge difficulties. And those who have excess savings are enjoying the benefits of these high interest rates. It is like a "wealth transfer from the poor to the rich".
Graph: American diners
As the graph above shows, the wealthy are doing well.
Graph: McDonald's same-store sales decline
And those with less disposable income are not doing so well.
Putting it all together
What do the three examples above have in common? Paying and receiving interest have produced diametrically opposite results - the poor are getting poorer and the rich are getting richer. That's the heart of the matter. Wealth and assets are being transferred from the weak to the strong.
Why does all this matter? Monetary policy is based on aggregate data. On average, everything looks fine and stable. However, one mode in that distribution is suffering badly. High interest rates benefit the other mode. So by keeping rates high and waiting for the average to weaken, the Fed is actually squeezing the weak more, not helping the strong. Viewed from that perspective, it's a pretty twisted approach.
Why do wealth disparities continue to grow? Because the way monetary policy is implemented exacerbates wealth disparities. This is not a thesis on the virtues of wealth redistribution, but in many major areas of our economic life, wealth disparities will continue to grow until we have some kind of collapse, debt relief, or other tail event.
Conclusion
In our view, the Fed should have cut rates in July.
Employment has peaked and is clearly falling.
Inflation is 2.5%, falling rapidly, and expected to reach the 2% target by year end.
However, real interest rates are currently 3%. In a steady-state, healthy economy, historically this number has been around 1%.
So what is the Fed doing?
They are focusing on the aggregate data and ignoring the underlying distribution.
This is where strategic errors occur.
The wealthy and cash-rich are enjoying higher interest income (not to mention assets are near all-time highs). The cash-poor are being hammered by interest expenses. Insensitive to, and even benefiting from, higher interest rates, the Federal Reserve is effectively waiting for the lower socioeconomic groups to deteriorate further in order to bring the average figures down to their target. Sorry, poor people, you are suffering with little benefit.
If the Fed allows "tight monetary policy" to continue (that's what they call it), they will face serious employment problems and the hollowing out of small businesses. Once this happens, history shows that it is difficult to reverse. They face the risk of a hard landing.
Everything seems normal until things suddenly go wrong. Change often happens slowly and then in a flash.
Original link
Disclaimer: The content of this article solely reflects the author's opinion and does not represent the platform in any capacity. This article is not intended to serve as a reference for making investment decisions.
You may also like
Will Kaspa (KAS) Bounce to Continue Its Rebound? This Fractal Says Yes!
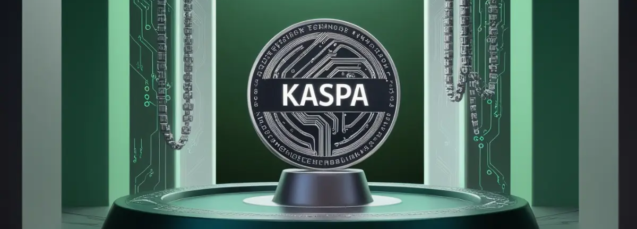
Is Bittensor (TAO) Repeating Its History? Fractals Hint at Continuation of Bullish Rally
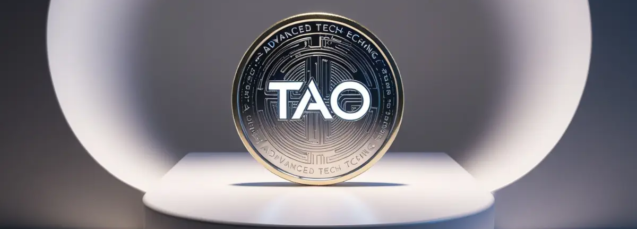
Will Injective (INJ) Bounce Back to Extend Its Rebound? This Fractal Pattern Says Yes!
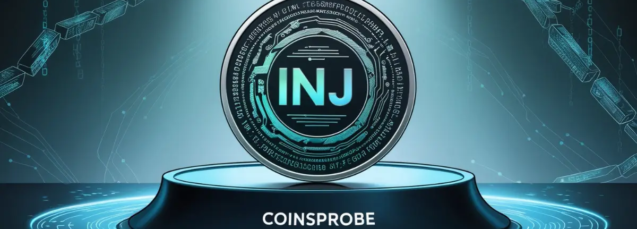
Can Cardano (ADA) Bounce to Continue Its Rebound? This Pattern Says Yes!
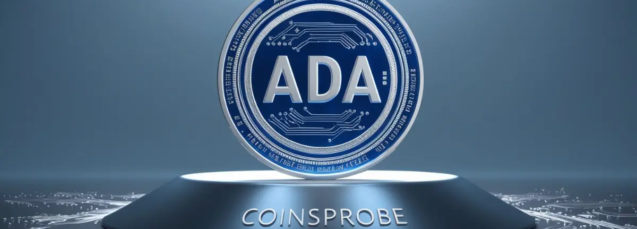
Trending news
MoreCrypto prices
More








